Towards in silico selection of interfacial actives: Discovery of new corrosion inhibitors for high value coating formulations
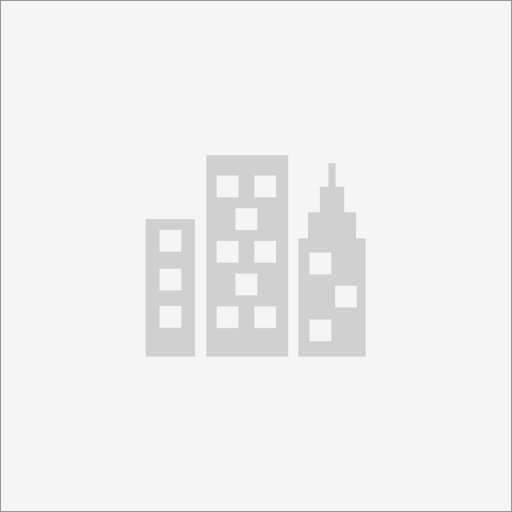
The University of Manchester
About the Project
The goal is to discover new corrosion inhibitors that can be added to coatings for protection of metallic infrastructure. Initially, you will quantify two key performance indicators for candidate molecules, i.e., corrosion reduction and diffusion through the coating matrix. Subsequently, you will use these data, combined with interfacial analysis results, as input for computational methods, including artificial intelligence (AI), that will allow identification of new highperformance species.
Project Description:
Coatings are key to optimising the properties of metallic substrates in a huge variety of realworld applications. For example, non-metallic coatings (paints) are widely employed to prevent corrosion in wet/humid environments, such as those experienced in the transportation and construction industries. Unfortunately, however, these coatings are not perfect barriers, and water can penetrate in time to the metal/coating interface, resulting in underpaint and localized corrosion phenomena. Moreover, these coatings are often scratched in everyday use, and any exposed metal can also corrode. Consequently, one cannot rely upon a coating’s barrier properties alone. Instead, so-called active corrosion control is also required, which is invariably achieved by embedding corrosion inhibitor pigments into a coating. Upon exposure to water, such species can dissolve and diffuse to the coating/metal interface and/or to scratches. Subsequently, they provide protection by retarding the anodic (metal oxidation) and/or cathodic (oxygen reduction) components of the electrochemical corrosion reaction. For decades, inorganic chromate pigments have been the actives of choice in coatings, providing unrivalled protection due to mixed anodic/cathodic inhibition. Concerns about the toxicity of chromium (VI) compounds, however, has culminated in increasingly strict regulation restricting their use. On this basis, there has been an immense effort directed towards the discovery of alternatives to chromate, although no silver bullet has yet been identified.
Of late, there has been increasing interest in using organic compounds as inhibitor pigments in coatings, which may act alone or in tandem with non-chromate inorganic pigments. Partially, this is driven by the successful use of such species for inhibition of metals in acidic environments, e.g., internal surfaces of pipes carrying oilfield/geothermal fluids. Expecting the same species to function similarly in a coating is, however, naïve, as the corrosive environment is very different, e.g., the pH is invariably highly alkaline, and the cathodic reaction is O2 reduction rather than H+ reduction. Furthermore, species also need to display suitable solubility/diffusion in the coating to be plausible actives.
Motivated by the above scenario, work is required to determine the most suitable organic corrosion inhibitor pigments for integration into coating formulations. Traditionally, this objective would be achieved by trial-and-error candidate testing, which is both financially expensive and time hungry. In this project, the intention is to massively speed up this process through harnessing AI, and related computational tools, to develop new in silico performance prediction capability using experimental data acquired from a carefully curated selection of candidate organic species. Assuming a successful outcome, this work will allow us to begin to efficiently identify new high performing corrosion inhibitors. These new actives will play a key role in lifetime extension of assets fabricated from metals, which will reduce the global carbon footprint associated with ore extraction, processing and fabrication; CO2 resulting from steel production to replace corroded infrastructure is expected to account for ~5 – 10% of total CO2 emissions by 2030. This project is well aligned to the CDT, as it will provide training for a PhD student in AI and related computational tools, along with relevant testing/characterisation techniques, targeting accelerated materials discovery. Moreover, it will promote the added-value of cross-disciplinary thinking/research, as computational approaches exploited for drug discovery (see below) will be reworked for corrosion control. Consequently, the research will drive cultural change for many corrosion researchers/engineers through demonstrating the power of in silico techniques for selection of high-performance actives.
Supervisors:
- Andrew Leach ([email protected])
- Suzanne Morsch ([email protected])
- Rob Lindsay ([email protected])
If you are interested in this PhD, we encourage you to contact the project supervisor(s) directly.
Application Deadline:
Applications open until successful candidate is recruited (no later than Summer 2025)
Funding Notes:
This is a fully funded project, part of cohort 2 of the EPSRC CDT in Materials 4.0. CDT. The studentship covers fees (home & international), a tax-free stipend of at least £19,237 plus London allowance if applicable, and a research training support grant.
Candidates of all nationalities are welcome to apply; up to 30% of studentships across the CDT can be awarded to outstanding international applicants. Early applications from interested overseas candidates are encouraged.
The Materials 4.0 CDT is committed to Equality, Diversity and Inclusion. Five countries are represented in cohort 1. We would like to see a more gender-balanced cohort 2, so we strongly encourage applications from female candidates.
Enquiries:
For application-related queries, please contact Paulina Hoyos ([email protected]). Please note that each partner of the CDT in Materials 4.0 will have its own application process.
Application Webpage:
https://pgapplication.manchester.ac.uk/apply/signon.html
Select Postgraduate Research, then 2025/26 academic year, and then CDT in Materials 4.0.
To help us track our recruitment effort, please indicate in your email – cover/motivation letter where (jobs-near-me.eu) you saw this job posting.