Robust, scalable statistical learning for dynamic network data
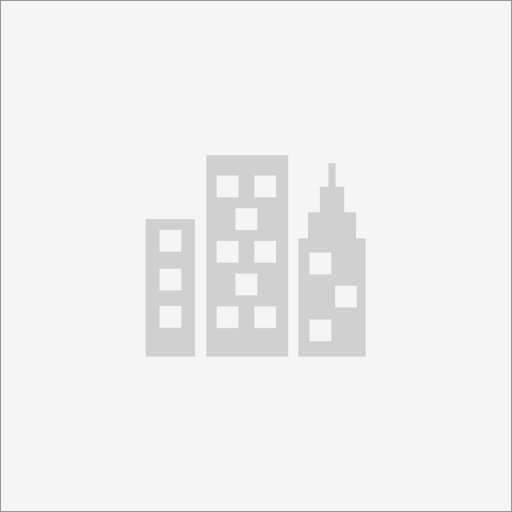
University of Bath
About the Project
This project is one of a number that are in competition for funding from the University of Bath URSA competition for entry in September 2025.
Overview of the Research:
There has been a recent explosion in the collection of network data, arising across a multitude of fields from biology and medicine to social media, cyber security and finance. In many scientific areas, the number of network entities can be large, and the characteristics of the network, or the data observed on it, can change over time. These aspects give rise to methodological and computational challenges when developing statistical techniques. To maximise the potential of such data, there is a need for mathematically rigorous analysis tools which are specifically designed to capture complex data dynamics in different scenarios.
A fully-funded 4-year PhD scholarship is available in the area of statistical learning for network-structured data. The successful student will contribute to developing statistical models of evolving, inter-connected stochastic processes by exploiting information at network nodes and edges. The intended applications of the framework include forecasting, detection of anomalous events and classification of node/edge states. We will use these new tools to derive impactful insight in a range of scientific and industrial applications.
This exciting opportunity is aligned to the strategic EPSRC-funded research programme on Network Stochastic Processes and Time Series (NeST) which brings together researchers at the Universities of Bath, Edinburgh, Oxford, York, Imperial College London and the London School of Economics and Political Science, with industrial and government partners such as BT, EDF and the Office for National Statistics.
The successful candidate will be working with Professor Matthew Nunes at the University of Bath node, be part of the growing NeST team, and engage in collaboration between institutions.
Candidates should be self-motivated, with a keen interest in developing methodological and computational statistical techniques to answer practical problems, backed up by demonstrably strong mathematical/statistical and programming skills.
Project keywords: statistical learning, network data, stochastic processes
Candidate Requirements:
Applicants should hold, or expect to receive, a First Class or high Upper Second Class UK Honours degree (or the equivalent) in Statistics, or a closely related field. A master’s level qualification would also be advantageous.
Non-UK applicants must meet the programme’s English language requirement prior to a formal offer being made.
Enquiries and Applications:
Informal enquiries are encouraged and should be directed to Professor Matthew Nunes, [email protected].
Formal applications should be submitted via the University of Bath’s online application form for a PhD in Statistics prior to the closing date of this advert.
IMPORTANT:
When completing the application form:
1. In the Funding your studies section, select ‘University of Bath URSA’ as the studentship for which you are applying.
2. In the Your PhD project section, quote the project title of this project and the name of the lead supervisor in the appropriate boxes.
Failure to complete these two steps will cause delays in processing your application and may cause you to miss the deadline.
More information about applying for a PhD at Bath may be found on our website.
Equality, Diversity and Inclusion:
We value a diverse research environment and aim to be an inclusive university, where difference is celebrated and respected. We welcome and encourage applications from under-represented groups.
If you have circumstances that you feel we should be aware of that have affected your educational attainment, then please feel free to tell us about it in your application form. The best way to do this is a short paragraph at the end of your personal statement.
To help us track our recruitment effort, please indicate in your email – cover/motivation letter where (jobs-near-me.eu) you saw this job posting.