Clear and sound: combining image analysis and bioacoustics to link pollinators traffic with fruit set and harvest
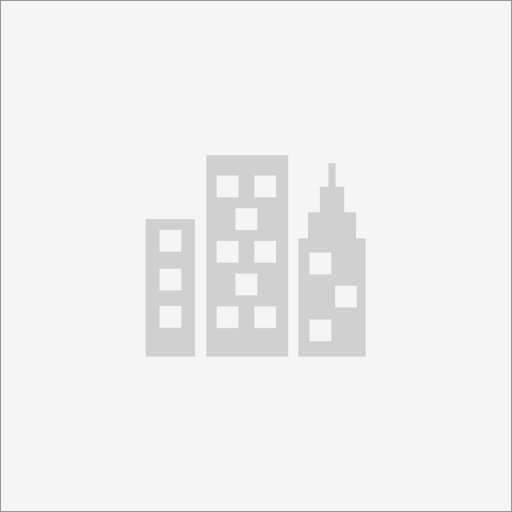
University of Aberdeen
About the Project
Insect pollinators provide fundamental ecosystem services to wild plants and commercial crops, promoting the transfer of pollen between flowers and across plants. Bees are some of the most important pollinators worldwide [1]: their biology and ecology are well known, and some species like Apis mellifera and Bombus terrestris are used commercially to foster the pollination of a wide range of crops, from open field oil seed rape to soft fruit in polytunnel or greenhouse setups. However, for many crops, we still lack details on how pollinators interact with flowers, as it is challenging to carefully record all pollinator-flower interactions, in particular when this is done in person by human observers. For this reason, the development of approaches for remote monitoring is a field that has received much attention in the recent past [2, 3] and has the potential to undergo a significant development in the near future. In this project, the student will make use of both camera and acoustic monitoring to carefully record all pollinator-flower interactions, as well as the levels of colony activity when bees are foraging. This large set of data will be processed with the support of machine learning tools developed by members of the supervisory team (Strathclyde and JHI) to identify how colony traffic affects flower visitation rates, and how these two measures link to successful fruit set and harvest. These experiments will be performed in both open fields and glasshouse/polytunnel setups available in the partner institution (JHI), targeting a range of pollinator species and suitable crops. The student will receive key training in a broad range of disciplines, from behavioural ecology and physiology of insects (Aberdeen), to bioacoustics [4], image analyses [5], and machine learning methods to process these data (Strathclyde, JHI and AgriSound).
Methodology
The student will carefully record all insect-flower interactions with Afidus ALT-200s cameras and bioacoustic recording devices (AudioMoth or in-field sensors): these devices will be placed near chosen patches or clusters of flowers for continuous monitoring. Different bee pollinators like honeybees, commercial bumblebees and mason bees will be targeted, and wild pollinators visiting flowers will also be recorded. Similar technology and approach will be used to monitor colony traffic, i.e. all movements of bees in and out of the nest. These observations will be complemented by transect walks to assess how remote monitoring reflects data obtained from in person recording. The large scale of data recorded over long time periods, in combination with the variability of acoustic and optical signatures from many different species of insect pollinators visiting plants simultaneously, make the data analysis an inherently difficult task. The project will investigate the use of machine learning to undertake classification and initial analysis of the acoustic and optical data sets. It will also explore deep learning models for analysis of the interrelationships between the acoustic and optical data and the fruit set and yield data. Foundational AI image models will be used to improve detection of pollinators in image data and innovative methods will be developed to classify species and behaviour of pollinators, incorporating current knowledge on the behavioural ecology of pollinators to improve accuracy.
Anticipated Outputs and Impacts
The comparative assessment of camera and acoustic data and their analysis in relation to fruit set and fruit yield data for the different setups will provide valuable information on how colony traffic influences flower visitation and, as a consequence, successful pollination and harvest. This output will be used to inform a range of stakeholders such as fruit growers, farmers, beekeepers and companies that distribute commercial pollinators: the supervisory team has already established connections with several of these parties on the occasion of a PhD studentship that is now in its third year. The output of this project will also allow for quantification of effectiveness of agricultural practices aimed to increase pollinator activity. Furthermore, findings from this research will be of interest for the commercial sector, in particular for business activities involved in the distribution of crop product. The CASE partner AgriSound works closely with major UK retailers like M&S and Tesco and will mediate the translation of this research into effective impact for UK customers.
To help us track our recruitment effort, please indicate in your email – cover/motivation letter where (jobs-near-me.eu) you saw this job posting.