Accelerated electrocatalyst design using artificial intelligence
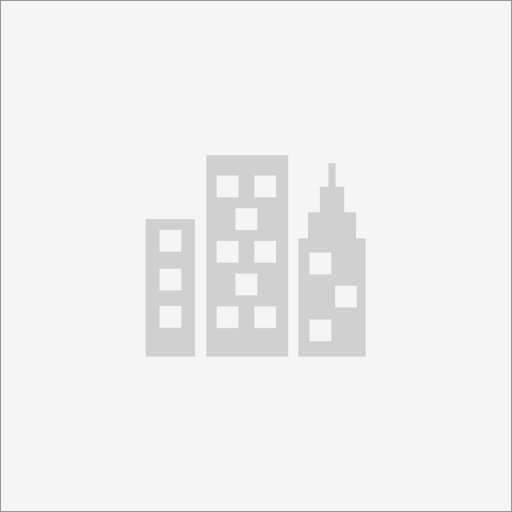
Imperial College London
About the Project
This project focuses on developing sustainable electrocatalysts for ammonia synthesis using artificial intelligence (AI). It will use multi-scale simulations to generate high-quality catalysis datasets and train generative AI models to design novel catalysts, improving electrochemical ammonia production from air, water, and renewable energy. This collaboration between Imperial College and Entalpic aims to accelerate the design and optimisation of clean energy materials.
Project Description:
Catalysis is vital for a sustainable future, particularly for applications like nitrogen fixation, which is critical for global food and energy security. Traditional methods of ammonia production, such as the Haber-Bosch process, are energy-intensive and environmentally unsustainable, accounting for approximately 1.3% of global greenhouse gas emissions. This process requires high temperatures and pressures, leading to the need for large, centralised facilities.
To address these challenges, we will advance the design and optimisation of electrocatalysts using artificial intelligence (AI), including property prediction and generative machine learning (ML) models tailored for electrochemical systems. Recent advances are given in [1,2]. Traditional methods for catalyst design are limited by their inability to accurately capture complex interactions across multiple time/length scales. Developments in materials modelling have provided valuable insights but are expensive and limited in their applicability to electrified solidliquid interfaces. Our approach leverages charge-aware ML force fields that can simulate electrocatalytic processes with greater efficiently and accurately. By modelling critical parameters such as crystal orientation and electrolyte interactions, we aim to capture a more comprehensive picture of the catalytic process.
We will focus on electrochemical nitrogen reduction as a sustainable alternative to the HaberBosch process. Li-mediated electrochemical synthesis has demonstrated the potential for decentralised ammonia production, potentially allowing for small-scale systems that operate at ambient conditions using renewable energy sources. Our recent research identified unique properties of Li that facilitate NH3 synthesis, such as stable nitride formation and ideal solidelectrolyte interphase [3]. We seek to extend these insights to explore alternative chemistries beyond Li. By understanding the features that contribute to efficient nitrogen fixation, we aim to develop catalysts that are more durable, efficient, and environmentally friendly.
ML force fields can accurately simulate complex electrocatalytic environments. By combining these with first-principles calculations, in a multi-scale approach building on our recent research for charged solid-liquid interfaces, we aim to explore the mechanistic pathways of nitrogen fixation and other catalytic processes. These approaches will be used to develop datasets on surface and interface evolution, as well as reaction pathways, under different applied potentials. The collaboration with Entalpic will provide access to cutting-edge data-driven AI architectures, enhancing our ability to predict and optimise catalytic behaviour. This includes using in-house ML force fields and generative models specifically designed for catalysis and active learning pipelines. We aim to validate computational predictions with experiments, through alignment with ongoing research within the group of co-supervisor Ifan Stephens (ICL). Experimental constraints will be integrated into our generative models to enhance the synthesis abilit of the predicted catalysts.
This research aligns with the CDT focus on data-driven materials design and sustainable energy solutions. The insights gained will not only advance the field of nitrogen fixation but also have broader implications for other catalytic processes, such as CO2 reduction. The findings will be translated into practical applications, driving innovation in catalytic technologies. We aim to pave the way for accelerated materials development cycles.
Supervisors:
- Aron Walsh (a.walsh@imperial.ac.uk)
- Ifan Stephens (i.stephens@imperial.ac.uk)
If you are interested in this PhD, we encourage you to contact the project supervisor(s) directly.
Application Deadline:
Applications open until successful candidate is recruited (no later than Summer 2025)
Funding Notes:
This is a fully funded project, part of cohort 2 of the EPSRC CDT in Materials 4.0. CDT. The studentship covers fees (home & international), a tax-free stipend of at least £19,237 plus London allowance if applicable, and a research training support grant.
Candidates of all nationalities are welcome to apply; up to 30% of studentships across the CDT can be awarded to outstanding international applicants. Early applications from interested overseas candidates are encouraged.
The Materials 4.0 CDT is committed to Equality, Diversity and Inclusion. Five countries are represented in cohort 1. We would like to see a more gender-balanced cohort 2, so we strongly encourage applications from female candidates.
Enquiries:
For application-related queries, please contact Jess Lewis (J.S.Lewis@leeds.ac.uk). Please note that each partner of the CDT in Materials 4.0 will have its own application process.
Application Webpage:
https://prod.banner.leeds.ac.uk/ssb/bwskalog_uol.P_DispLoginNon
Select research degree – research postgraduate, then 2025/26 academic year and, under ‘planned course of study’, choose ‘EPSRC CDT Materials 4.0’.
To help us track our recruitment effort, please indicate in your email – cover/motivation letter where (jobs-near-me.eu) you saw this job posting.